How AI undermines academic norms
Do standard practices ensure high university standards anymore?
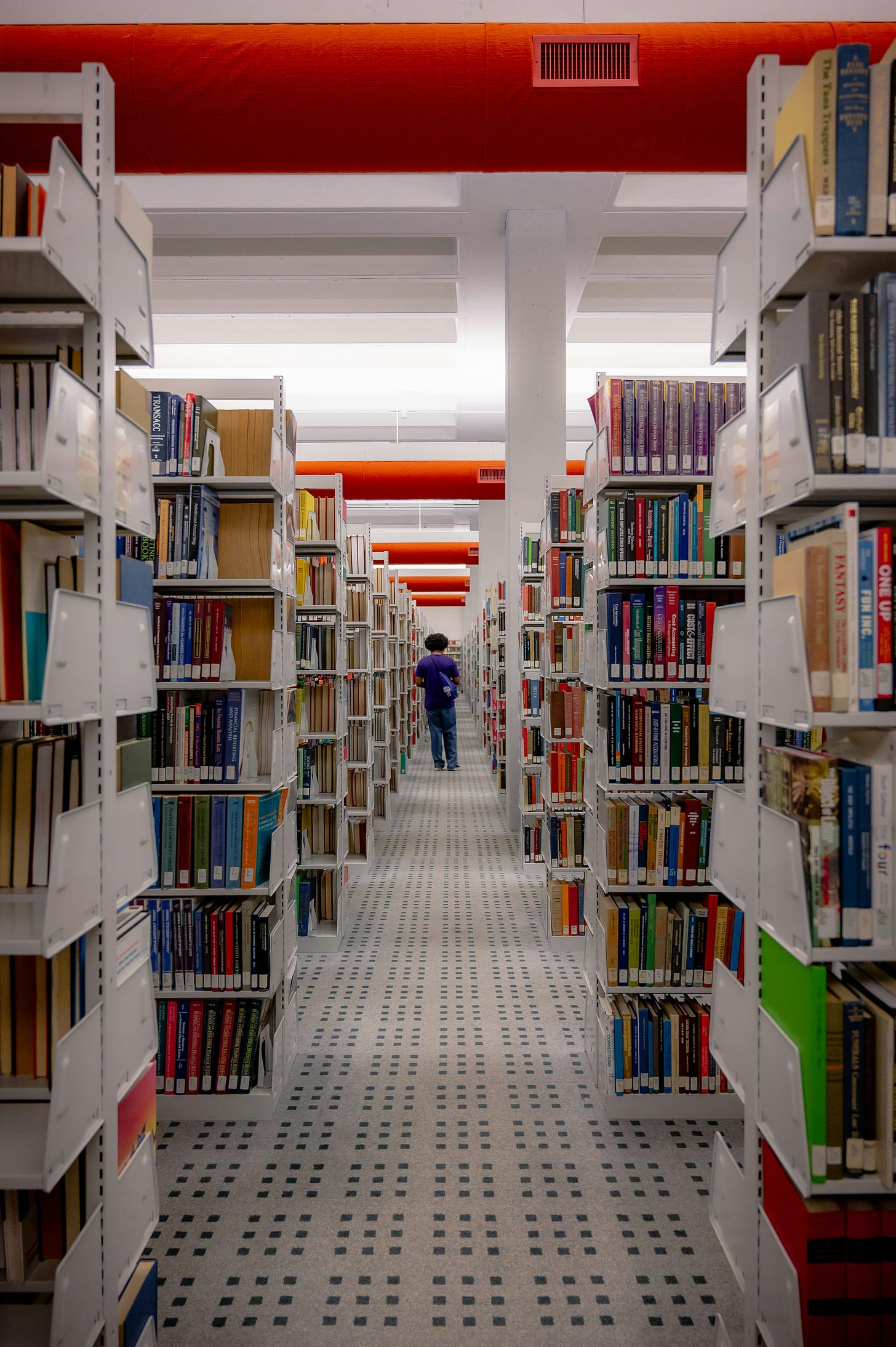
Last year, as part of my day job, I wrote a report about future disruptions for universities. It was commissioned by a major Australian review into our university sector and was recently made public. Unsurprisingly, the potential impact of modern AI was a significant theme of the report. However, after my most recent post here, I've realised that I didn't really get to the heart of some important issues.
In the report, I noted that "The ability of [generative AI] programs to produce reasonable quality academic work on any topic challenges traditional approaches to teaching and assessment." (p.9) As has been widely noted, we have well-established methods of identifying the quality of a student and the skills they are learning, like exams and essays, that are now under question as AI can, and perhaps should, replace some of them. These challenges aren’t restricted to academic teaching - as similar issues are arising in academic research. If AI can write, and peer-review, academic articles to a reasonable standard, how do we assess and guarantee the quality of research?
Modern academia has a wide range of habits and measures that exist to incentivise and ensure we get high quality teaching and research. These range from norms around assessment and marking, to accepted writing styles and peer review of research, to standards for research funding applications, citation metrics, student feedback and evaluations, research quality and impact assessments, and so on. However, a conclusion from my recent post on AI has lead to a suspicion that many of these might be assessing the wrong things.
In that post, I argued that AI is teaching us that some skills we took as demonstrating the highest levels of intelligence, like playing chess, only happen to be hard for humans, not for machines. On the other hand, many skills that we take for granted, like object identification, demonstrate high levels of distinctly human intelligence. If we have been looking in the wrong places for evidence of distinctive human intelligence, then it would not be surprising that we have been using the wrong assessment and quality approaches in academia. Assessing human skills against what AI can do can help make this clearer.
Proxies for the real thing
Across both teaching and research, universities have historically expected people to demonstrate their skills at particular types of activity and we then take these as evidence of the underlying qualities we really want. We get students to sit exams so we know that they have mastered a body of knowledge. We ask students to write essays so we know they can think clearly and carefully. We expect researchers to write in a careful, considered, neutral style as we want them to put aside their own preferences and pursue genuine knowledge. We ask academic peers to review and pass research before publication, as we want it to stack up as good quality in any given field.
Many of the distinctive practices of academia are, in the end, easy to implement proxies for what we were really looking for. They have been sufficiently rigorous and useful that they are strongly embedded and many cannot imagine a university without them. Often the proxies end up being treated as the end goals. The aim of many researchers is to publish peer-reviewed articles, whatever it takes, rather than publish good quality and valuable research that happens to be verified by peer-review.
There have always been problems with these proxies, but modern AI is making them very clear - as generative AI can deliver the desired outputs better than most humans, but without necessarily demonstrating the underlying qualities we are looking for. In other words, our proxies are no longer fit for purpose as measures of important skills or attributes.
For example, in the past, if you received a well written, grammatically accurate, polished essay that was clearly structured and argued, it could be taken as evidence that the person who wrote it was capable of thinking clearly and making informed judgements. Today, if you receive a highly polished essay with sophisticated grammar, you could just as safely assume that someone used generative AI to write it - and it is unclear what it says about anyone's skills.
The same issues are emerging across all areas of academia: it's hard to distinguish whether someone is across a body of knowledge or can just use an internet search; a considered academic style of writing just as often hides clear biases as removes them and is easily repeated by AI; not only are people using generative AI to conduct peer review but it is unclear what it demonstrates even when done by humans.
It is therefore, at the very least, up for debate whether the existing norms and practices of academia are fit for purpose. Core assumptions about how university teaching, training and research should work are being called into question, and may need to be rethought. Universities are rewarding people for delivering certain outputs that no longer seem to reflect the underlying skills or qualities that we actually want.
To pick one example, we ask researchers to publish lots of peer-reviewed papers - and the number of peer-reviewed papers being published has rapidly increased, yet there is a widely acknowledged slow down in the development of new knowledge and research productivity.
Redesigning academia
If universities are relying on practices that no longer deliver the desired value or results, they will become increasingly irrelevant. Consistently decreasing enrolments in some countries suggests this is becoming an issue. However, universities have existed for centuries despite huge societal changes for good reasons. They may need to, once again, rethink how they operate to continue in importance.
I don’t have a clear road map but, as you would expect from a philosopher, strongly believe we need to go back to first principles. That is, we need to be clear about what it is we really want or value - and then assess whether current approaches still work. For example, if we value new, reliable knowledge, then measuring the number of papers written - including citations - will be increasingly unhelpful, especially as AI will accelerate the number of publications and citations without necessarily accelerating the growth of knowledge. Or if we value students learning clear thinking and good judgement, then simply writing good essays is increasingly not going to be a good measure when AI will be used to do that work.
The ultimate purpose or desired skills will likely vary across fields, so there aren’t easy universal answers. And we especially need to think clearly about what it is exactly we want humans to be able to do, that we do not want to leave to AI or cannot leave to AI. I would suggest that identifying truth, making good judgements, understanding dynamics in the real world, and sense-making under ambiguity are some of the skills we will always need humans to be responsible for.
We then need to figure out how to measure the things we really value for humans - and what that looks like today. It may look old school - like handwritten exams or oral defences - or may be very modern - like demonstrating effective prompt engineering to ensure AI gives accurate answers. It might be that we can let go of some of our measures - perhaps peer review is no longer relevant and we can rely on the public review that currently occurs on pre-print servers - or we need to replace them with something more rigorous - academic publication might need to run through an antagonistic process like a 'red team' before publication. It might be less involved and cheaper than current practices - perhaps a one off set of cases and interviews can establish whether someone has better thinking skills and judgement than 3 - 5 years of classes - or it might be far more intensive and expensive - rigorous, one-on-one tutoring might be the way forward.
Stopping and taking time to be clear about qualities or skills we need in universities and the best ways to achieve them today might sound fairly simple, but will be incredibly hard to do in practice. Universities are big and highly regulated organisations that have specialised for a long time in delivering particular outputs (degrees, academic papers, etc). Change is always hard. But without it, academia will no longer deliver what broader society expects from it.
Finally, I should note these problems aren’t unique to universities. Many fields are facing the same sort of challenges in the face of AI - as what we take as guarantors of quality are no longer fit for purpose.
Well put. Speaking of other fields: I work on software engineering and measuring the candidates' abilities in recruitment has long been a hard problem. (Google did some interesting research showing that nothing seemed to work very well...) But the ability to write a clear CV and cover letter with good grammar and that addressed the job ad has been used as a proxy for communication skills and I think that is on the edge of obsolescence due AI. The ability to do short coding puzzles is another key proxy and doing them offline (as "homework") became increasingly popular both due to Covid and other issues with doing it as an in person exam. Just last week I wrote a recruitment challenge and was quite disturbed how well ChatGPT did with it. The LLM coding is long way from good enough to actually replace the engineer but it's hard to work out what the new proxy could be.
Now be honest. Could it be that you had some artificial help writing this piece? Either way, an excellent analysis. Your phrase 'distinctive human intelligence' is worthy of a deeper dive sometime in the future. Of course, intelligence is not the only 'human' trait we value.
I wonder also if AI is reducing what Daniel Kahneman called noise (or to use another word, diversity) in output. This has a positive side which is obvious - outputs are, on average, better. This is largely because they are more consistently a bit better than average. There is, however, in my mind anyway a negative. My argument would be that knowledge creation requires diversity. Good knowledge building systems are able to identify things that are both novel and better (or truer if you prefer). For this to happen, you need outputs that challenge the average rather than simply propagate it. Otherwise, we are accepting that best lies somewhere in a tight distribution around the average.
All of this raises a question for me around the difference between exploration and application. I am wondering whether, as humans, we need to identify more clearly where we are seeking to apply existing knowledge (even in writing an essay) or explore new knowledge.